Potential Outcomes Model (or why correlation is not causality)
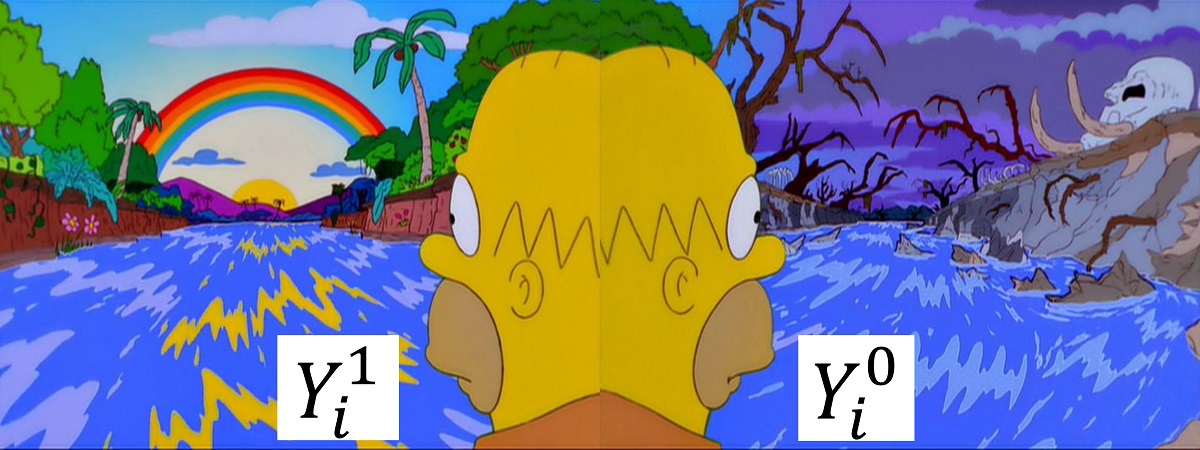
This article, the second one of the series about the book Causal Inference: The Mixtape, is all about the Potential Outcomes notation and how it enables us to tackle causality questions and understand key concepts in this field1.
The central idea of this notation is the comparison between 2 states of the world:
The actual state: the outcomes observed in the data given the real value taken by some treatment variable.
Introduction to causal diagrams (DAGs)
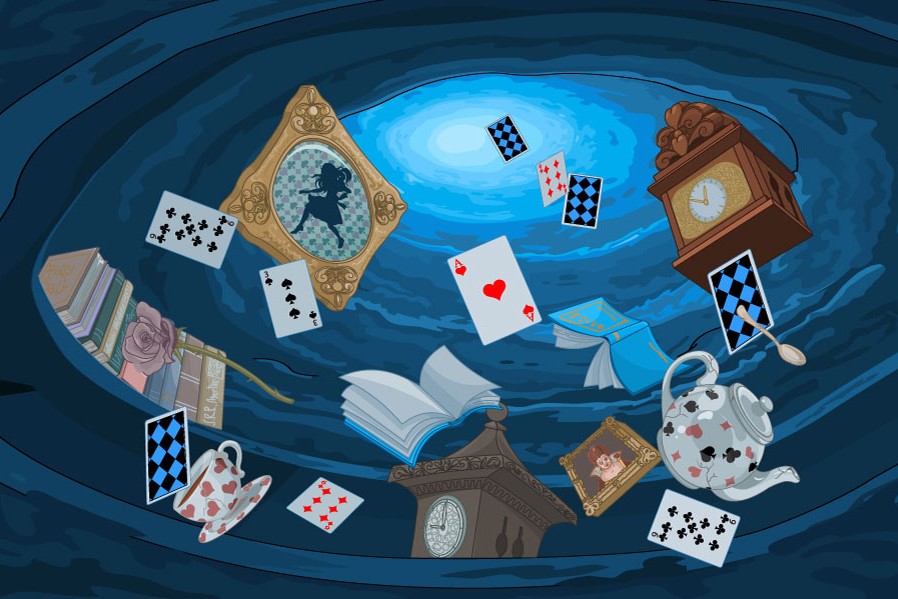
This article is the first in a series dedicated to the content of the book Causal Inference: The Mixtape, in which I will try to summarize the main topics and methodologies exposed in the book.
DAGs (Directed Acyclic Graphs) are a type of visualization that has multiple applications, one of which is the modeling of causal relationships.
We can use DAGs to represent the causal relationships that we believe exist between the variables of interest.