Building an Airflow Pipeline That Talks to AWS — Data Pipelines in the Cloud (III)
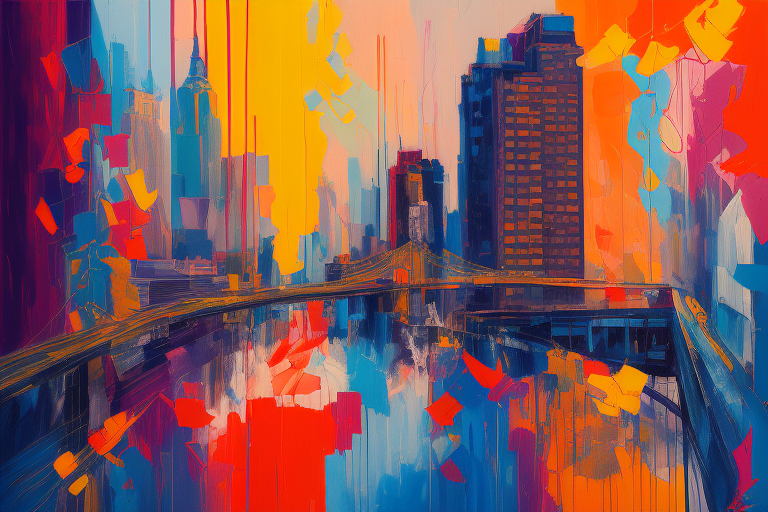
This tutorial is a complete guide to building an end-to-end data pipeline with Apache Airflow that communicates with AWS services like RDS (relational database) and S3 (object storage) to perform data transformations automatically and efficiently.
Using Amazon Web Services (AWS) with the Command Line — Data Pipelines in the Cloud (II)
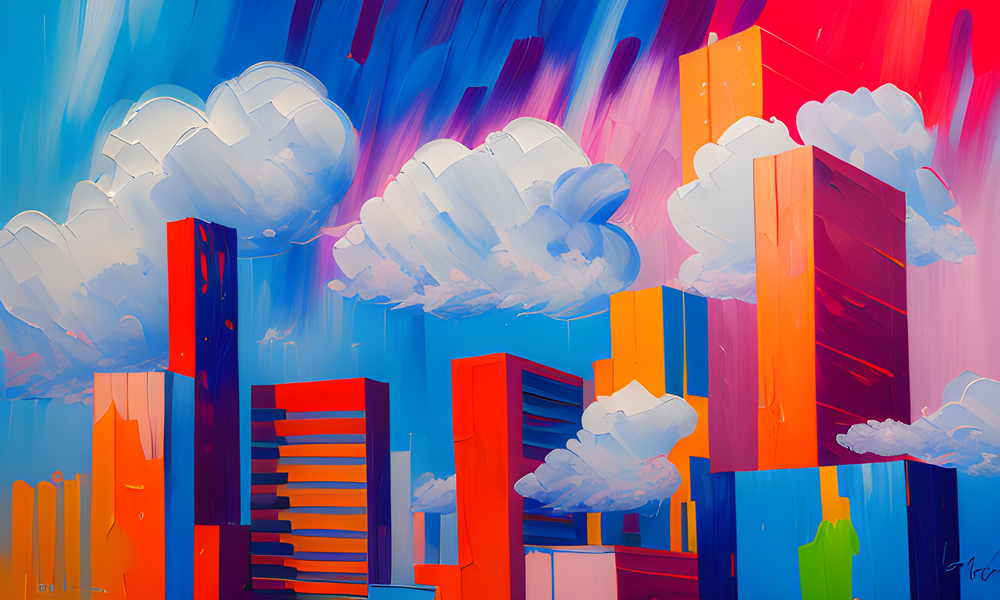
Welcome back to the ‘Data Pipelines in the Cloud’ series! In the first part, I introduced Airflow as a tool for orchestrating data pipelines and demonstrated how to code and execute a minimal Airflow pipeline (DAG) on your local environment. In this second part, we’ll lay the ground to build a more functional Airflow DAG by using the AWS Command Line Interface to set up a relational database in the cloud (PostgreSQL), along with a bucket for object storage (S3). We’ll then upload a sample CSV file to the bucket, which we’ll later use as input for an Airflow DAG that performs a meaningful transformation on this data.
Sharing My Advent of Code 2023 with Quarto (And How You Can Do the Same)
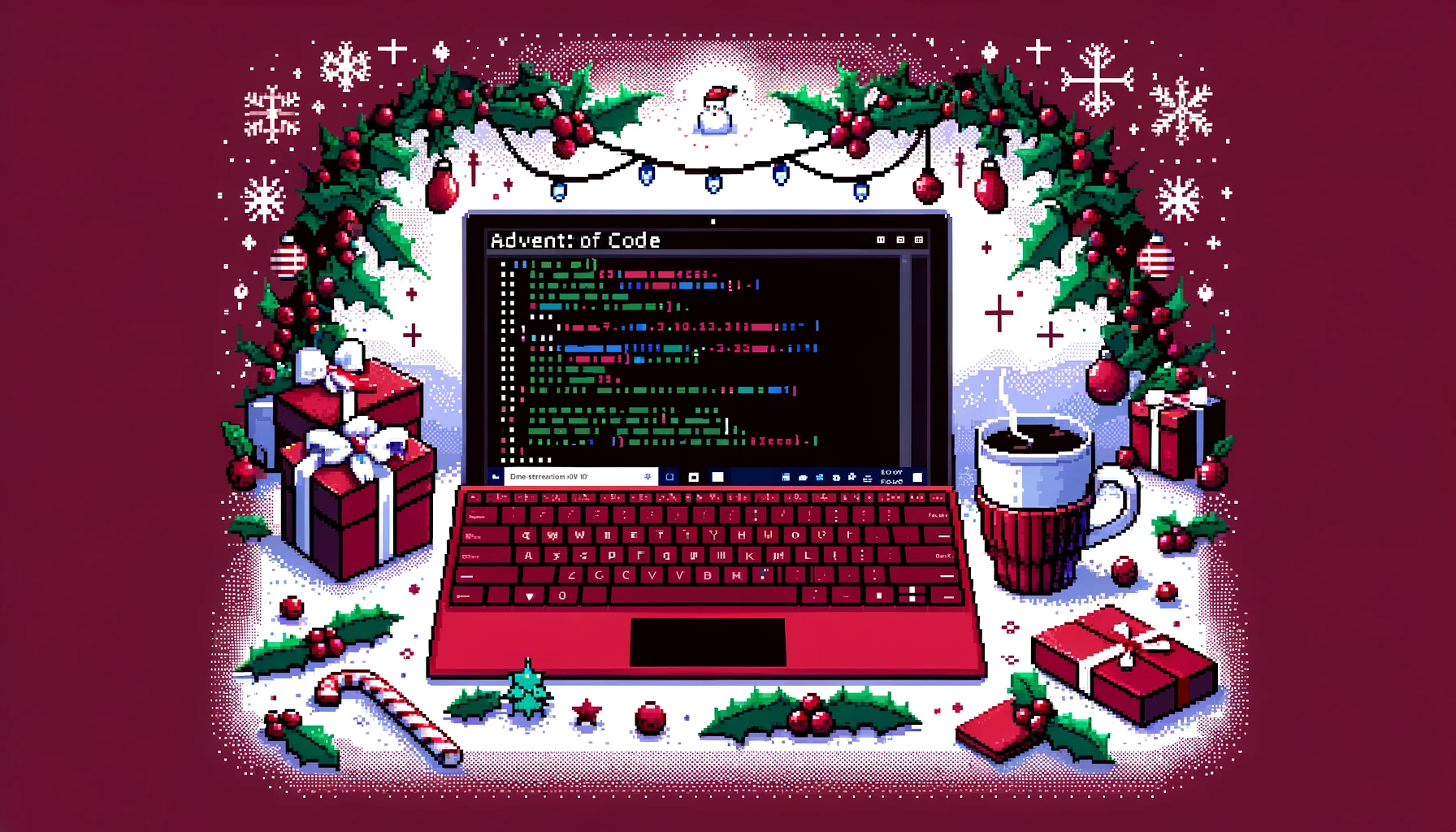
As a Christmas enthusiast, I’ve always been intrigued by the Advent of Code, a series of daily programming puzzles leading up to Christmas. This year, I’m taking on the challenge with either R or Python, adding a touch of whimsy by using a spinning wheel to choose my language each day. I’m also sharing my solutions on a special Advent of Code-themed website. Find out how you can create your own Advent of Code site and automate the process with the
aochelpers
R package.
A Beginner’s Introduction to Airflow with Docker — Data Pipelines in the Cloud (I)
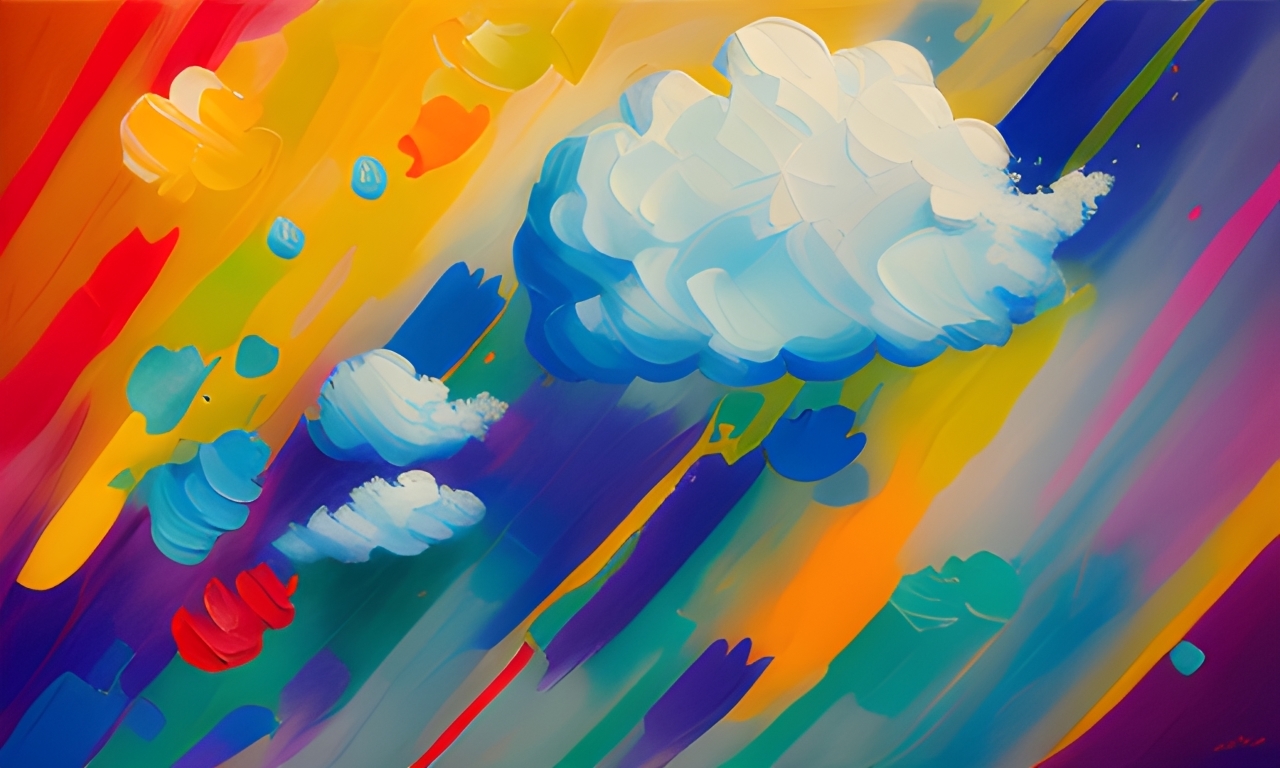
Learn the essentials of Apache Airflow for creating scalable and automated data pipelines in the cloud with this comprehensive, step-by-step beginner’s guide. Discover what problem Airflow solves and under what circumstances is better to use it and run your first Airflow DAG on Docker with the Linux subsystem for Windows.
Randomization Inference in R: a better way to compute p-values in randomized experiments
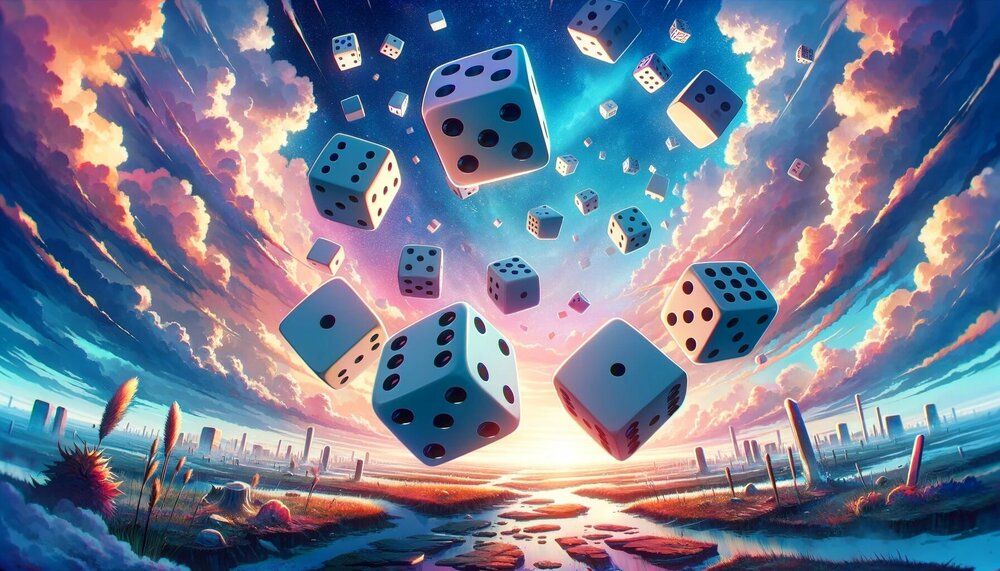
Welcome to a new post of the series about the book Causal Inference: The Mixtape. In the previous post, we saw an introduction to the potential outcomes notation and how this notation allows us to express key concepts in the causal inference field.
One of those key concepts is that the simple difference in outcomes (SDO) is an unbiased estimator of the average treatment effect whenever the treatment has been randomly assigned (i.